Science at work 4 March 2024
- Home
- CIRAD news
- News
- Predicting mango production - Sahel
Towards an app to predict mango production in the Sahel
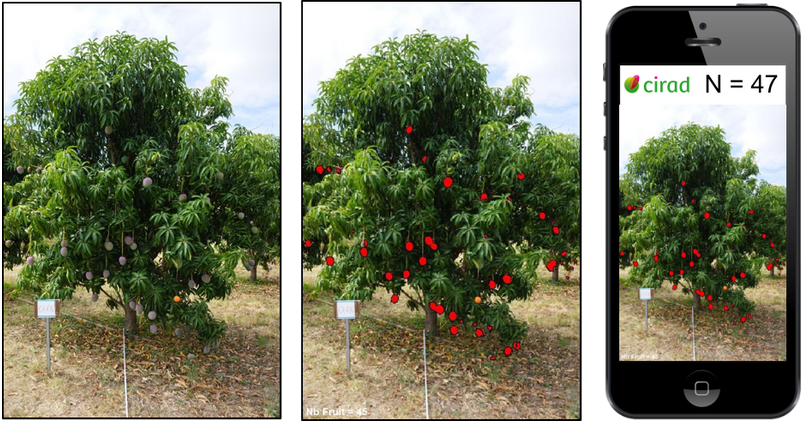
“One of the openings for my research is a smartphone app to help mango farmers estimate their future yields ”, says Julien Sarron, a PhD student in CIRAD’s HortSys research unit. Predicting mango yields is in fact the subject of the thesis, labelled #DigitAg, that he is working on in Senegal.
Useful information for all actors in the sector
Estimating agricultural yields prior to harvesting gives small farmers negotiating power during the sale of their products and enables exporters to better organize their logistics. Estimation tools already exist for the production of the major annual crops, but are more difficult to implement for perennial crops. This is the challenge taken up by Julien Sarron and his colleagues.
Understanding yield variability
The PhD student focused on mango orchards in the Niayes region in the west of Senegal. The main challenge was to adapt to yield heterogeneity at the individual tree level as well as at that of the orchard. Indeed, the yield from a plot varies according to geographical situation, planting density, agricultural practices, etc. And within the orchard, the mango trees will give more or less fruit depending on their age or their variety.
Modelling and image recognition
Julien Sarron and his colleagues at CIRAD and the Institut Sénégalais de Recherche Agricole (Senegal Agricultural Research Institute) developed a digital toolbox capable of quantifying production at both the tree level and the orchard level. These tools are based on modelling and artificial intelligence for image recognition (see box). The photos are taken with a simple digital camera in order to estimate production at the tree level, and with a drone to assess yields at the orchard level (see photos below). In 2017, this methodology passed the test of comparison with real yields, thereby demonstrating its effectiveness. The researchers are now considering extending their method to other perennial crop sectors such as cocoa, lychees and citrus fruits.
An application for farmers
Building on this success, Julien Sarron and his team are planning to develop a smartphone app. To implement this project they have requested support from the Société d’Accélération du Transfert de Technologies (SATT - Technology Transfer Acceleration Company) AxLR . “In order to obtain an accurate yield estimate, a farmer will just need to take a few photos of his mango trees and to enter information about his plot, such as the number of trees and the variety ”, says the young researcher. “This data will then be added to an online database also containing information on the climate, soil, administrative limitations, etc. The database will make it possible to spatialize yields. It will be available to all actors in the sector, buyers, exporters, local authorities, states, etc. ”. In terms of science, it will enable researchers to study the parameters that generate yield heterogeneity in and between orchards.
When deep-learning counts fruit
The image analysis system jointly developed by researchers from the HortSys and AMAP research units is based on a deep neural network specialising in mango recognition. Using a simple photo, the detection algorithm counts the number of fruits on a tree in just 0.3 seconds and with an error margin of 5%! This artificial intelligence system (deep neural network) was trained and calibrated on a set of more than 7200 annotated images of mango trees. It is thus highly robust, but also adaptable, since it works even for green fruits and for different mango tree varieties.